Researchers propose novel reconstruction method that trains artificial neural network with normal-dose CT images.
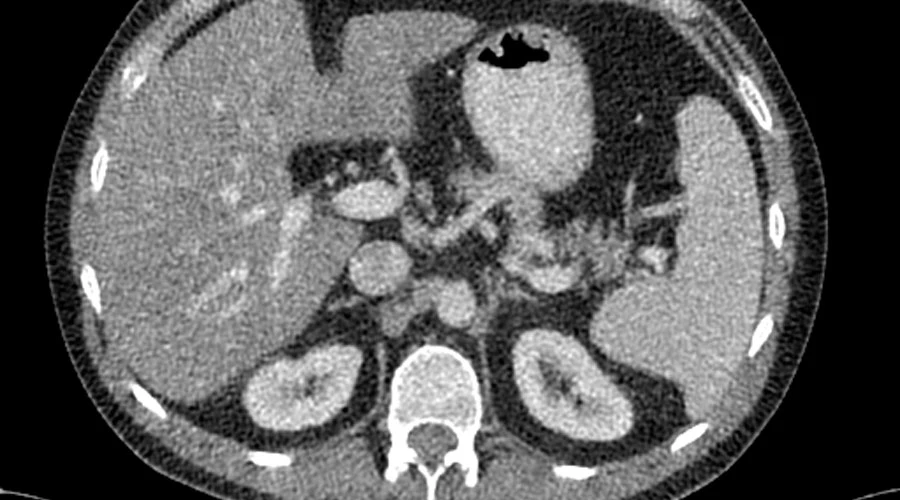
news from:
https://bioengineeringtoday.org/imaging/modeling-artificial-neural-netwo…
Four years ago, the U.S. Preventative Services Task Force published its recommendation that physicians should screen high-risk adults for lung cancer annually with low-dose computed tomography (CT). Compared to a conventional CT, getting a low-dose CT scan involves five times less exposure to ionizing radiation. However, compromised image quality remains the biggest barrier in completely replacing conventional CT with its low-dose counterpart. Decreasing the X-ray tube current leads to less radiation exposure, but also contributes to greater levels of noise and artifact in the resulting image. A recent study published in IEEE Transactions on Medical Imaging proposes a novel iterative reconstruction method that employs an artificial neural network (ANN)—a type of computational process inspired by biological neurons—to improve low-dose CT image quality. Researchers from Massachusetts General Hospital and Harvard Medical School trained the ANN on existing normal-dose patient CT images so it could learn what proper images are supposed to look like. “Nowadays, there are applications for CT that are not just diagnostic. There are some applications like screening for early cancer detection where you don’t want to use a normal CT dose,” said first author Dufan Wu, a postdoctoral research fellow at Quanzheng Li’s lab at Massachusetts General Hospital. “That is where low-dose CT comes in—but we want to improve the image quality to increase the accuracy and prevent misdiagnosis.” While the more common filtered back projection method directly calculates the image in a single step, iterative reconstruction techniques repeatedly update the image for a more precise result. Many include input called prior information, which was typically structural features such as boundaries the image should have. More recently, including the current study, scientists have experimented with machine learning to create prior information. The idea is to have an algorithm learn the most useful features that contribute to high-quality images. The reconstructed images are then processed to fulfill the requirements given by the neural network. To test the new reconstruction method, Wu, Li and their colleagues used data from the 2016 Low-Dose CT Grand Challenge, a competition designed to improve the quality of images from low-dose CT scans. The datasets contained quarter-dose chest and abdominal scans from 10 patients, simulated from the normal-dose data by noise injection. After using a training set of data for unsupervised learning, the ANN method achieved better performance for quarter-dose data than two other state-of-the-art iterative image reconstruction techniques, called dictionary learning and total variation. The ANN had greater noise suppression and structural preservation, maintaining acceptable performance with as low as one-sixth the normal dose. “The emerging trend is to train aspects of the model from good quality data, and then apply the trained model to reconstruct images from lower dose scans,” said Jeffrey Fessler, a professor of electrical engineering and computer science at the University of Michigan who was not involved in the study. “I think we are still in the early days of figuring out how to make good use of training data in such algorithms.” Fessler thinks the neural network method represents a useful step in the field and noted that many different research groups (including his own) are pursuing different variations on the same technique.