The new study, conducted in collaboration across multiple institutions, lays out a generalist medical AI model, BiomedGPT, to support multiple potential clinical applications. By evaluating on 25 datasets across 9 biomedical tasks and different modalities, BiomedGPT achived 16 state-of-the-art results. The human evaluation of BiomedGPT on three radiology tasks exhibits model’s robust prediction ability with the satisfactory error rates. Their results are published in Nature Medicine (https://www.nature.com/articles/s41591-024-03185-2)

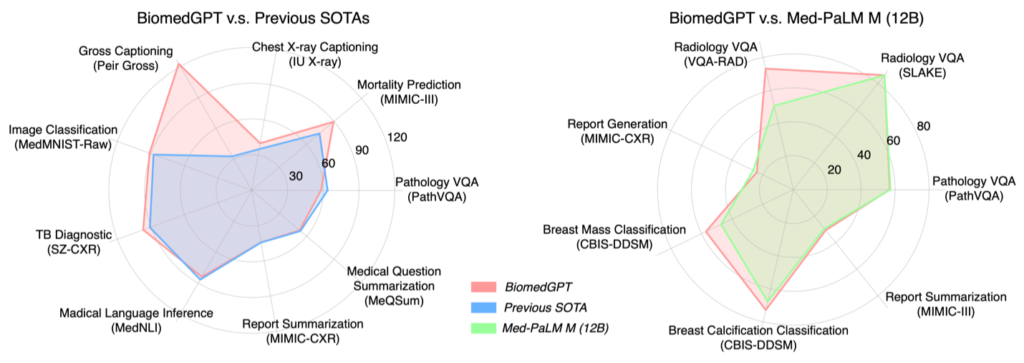
Unlike traditional medical AI models that are designed for specific tasks or modalities and can only address narrow aspects of the clinical puzzle, we are now entering a new era where generalist medical AI models like BiomedGPT are paving the way. These models are versatile in interpreting different data types and generating tailored outputs for diverse needs. Additionally, BiomedGPT is a fully open-sourced (code repo: https://github.com/taokz/BiomedGPT) and lightweight foundation model, making it accessible to researchers and practitioners for further customization.
BiomedGPT is implemented with a BERT-style encoder and a GPT-style left-to-right autoregressive decoder. These models rely on the transformer architecture with a multi-head attention mechanism, which enables the model to jointly attend to information from different data types, all of which are tokenized into a unified vocabulary. To enhance its generalist capabilities, BiomedGPT is pre-trained on a curated large-scale corpus comprising 592,567 images, approximately 183 million text sentences, 46,408 object–label pairs, and 271,804 image–text pairs across 19 different modalities. With well-trained vision-language representations, computing-friendly fine-tuning (continuing training on the pre-trained model) on the target dataset can achieve promising results. For example, BiomedGPT has achieved the state-of-the-art results on 16 out of 25 experiments regrading medical visual question answering, report generation & summarization, image-based diagnosis and lesion detection. Surprisingly, although BiomedGPT has only 182 million parameters—3,088 times fewer than the commercial generalist biomedical AI model Med-PaLM M—it still achieved comparable performance! Furthermore, BiomedGPT can perform zero-shot predictions on new data and answer medical questions in a freeform manner at scale, without requiring re-training. It also achieved the best accuracy on the VQA-RAD dataset compared to OpenAI’s powerful commercial model, GPT-4V. BiomedGPT has also been comprehensively evaluated by the medical professional at Massachusetts General Hospital (MGH) on three tasks in radiology, and it exhibits robust prediction ability with a low error rate of 3.8% in question answering, satisfactory performance with an error rate of 8.3% in writing complex radiology reports, and human-level summarization ability.
Although the results highlight BiomedGPT’s promising potential in medical applications, substantial enhancements are required to make it usable in clinical settings. Additional evaluations are particularly needed in the areas of safety, equity, and bias. The findings from this study underscore the challenges that must be addressed before a generalist medical AI model can be effectively deployed in clinical environments: First, access to diverse modalities and high-quality annotated medical data is critically important for developing the next generation of generalist medical AI models—those with more powerful conversational abilities, significantly reduced hallucination, and enhanced complex reasoning capabilities. Unfortunately, such data is still lacking for most researchers. Additionally, considering the time-consuming nature of human evaluations, the development of better automatic evaluation metrics, especially for freeform tasks like radiology report generation, is helpful for advancing the field.