Our paper, “Distribution-aware Fairness Learning in Medical Image Segmentation From A Control-Theoretic Perspective,” co-1st authored by Yujin Oh and Pengfei Jin, with Xiang Li and Quanzheng Li as corresponding authors from CAMCA, has been accepted to 42th International Conference on Machine Learning as a Top-2.6% Spotlight Paper!
Biases in medical image segmentation arising from demographic attributes (e.g., age, sex, race) and clinical factors (e.g., disease severity) remain a persistent challenge. We propose Distribution-aware Mixture of Experts (dMoE), a novel approach inspired by optimal control theory, designed to address heterogeneous data distributions and enhance fairness in medical image analysis. Our approach demonstrates robustness across diverse datasets—including two public 2D benchmarks and a proprietary 3D radiotherapy target delineation dataset—advancing the development of equitable and reliable AI-driven healthcare solutions. We’re excited to share this step toward fairer, more equitable AI applications in healthcare.
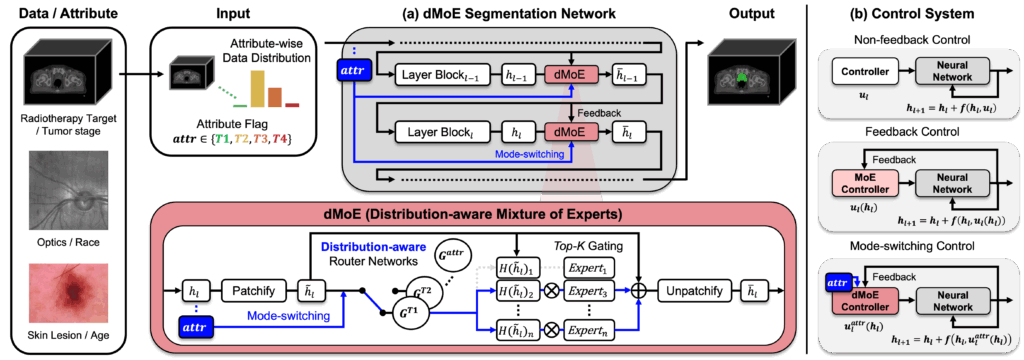